Pinecone AI Introduces Serverless Vector Database for Knowledgeable AI
The release opens up new possibilities for AI developers and researchers seeking to enhance the capabilities of Large Language Models through innovative vector database solutions.
Pinecone AI, a leading player in the AI tech stack, has unveiled Pinecone Serverless, a vector database designed to cater to the evolving needs of AI in the present era. Large Language Models (LLMs) have become the cornerstone of the AI tech stack, and to truly enhance their utility, they require access to comprehensive knowledge. Pinecone Serverless is positioned as a pioneering solution to address the knowledge gap in the AI domain.
Over the past year, a new AI tech stack has emerged, with Large Language Models serving as the intelligence and orchestration layer. Pinecone Serverless seeks to redefine vector databases, which are crucial for providing knowledge to LLMs and the broader AI stack. The development of Pinecone Serverless is the result of over a year of active development, aiming to solve critical challenges and pave the way for the future of vector databases.
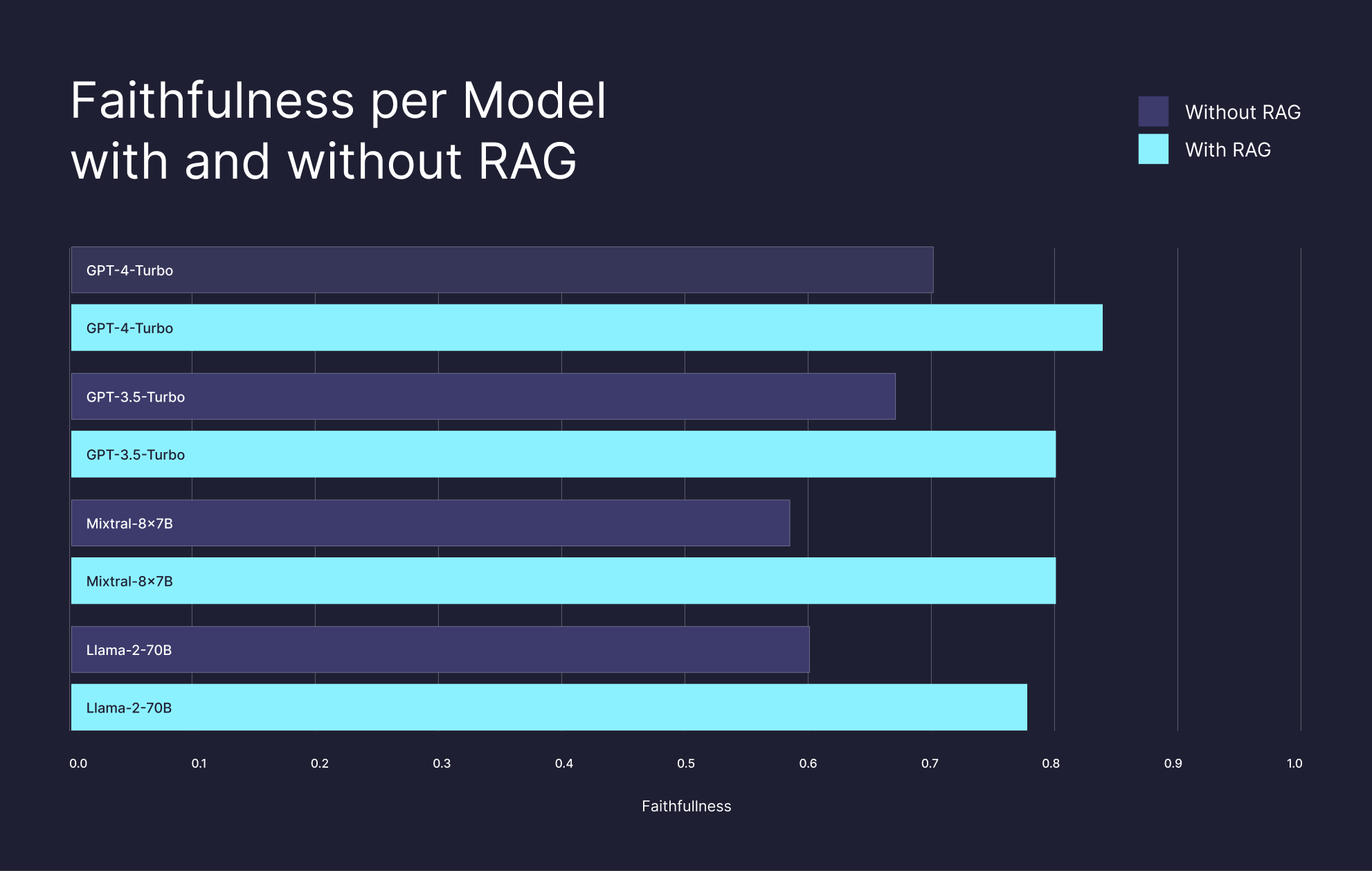
One of the key factors influencing the development of Pinecone Serverless is the diverse range of client use cases. An example highlighted is Gong, a client using an "active learning system" called Smart Trackers. This system employs Pinecone to process user conversations, embedding them into vectors for efficient searches. Notably, the on-demand nature of labeling in this use case required cost-efficient searches over billions of vectors, a challenge Pinecone Serverless effectively addresses.
Key Features of Pinecone Serverless
- Decoupling Storage from Compute: Pinecone Serverless tackles the challenge of decoupling storage from compute, allowing relevant portions of the index to be paged on demand from low-cost persistent storage. This is achieved through a separation of storage and compute, enabling cost-effective searches over large datasets.
- Multitenancy with Namespaces: To address the diverse usage patterns of individual users within a database, Pinecone Serverless introduces the concept of namespaces. Namespaces act as hard partitions, providing data isolation between tenants and supporting efficient queries with low latencies.
- Retrieval Augmented Generation (RAG): Pinecone Serverless enhances LLMs with knowledge through Retrieval Augmented Generation (RAG). By leveraging vector databases, it ensures that LLMs are equipped with precise and relevant information, reducing the likelihood of hallucination and enabling verification of information sources.
- Freshness, Elasticity, and Cost at Scale: Pinecone Serverless addresses the high demands for freshness, elasticity, and cost efficiency in vector databases. The architecture allows for incremental updates to the index, efficient scaling, and provides an up-to-date view of the index, essential for various AI applications.
Pinecone Serverless is released in public preview, offering users up to 50x cost savings and a novel vector database. The future roadmap includes expanding to more clouds and regions, introducing a performance-focused mode, and enhancing database security. Pinecone also plans to release a benchmarking suite along with methodologies for benchmarking vector databases.